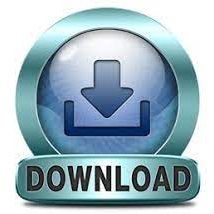
The first problem is that much of the literature has focused on elite runners. There are reasons to believe that both sets of questions – factors related to performance and race time prediction – have been poorly addressed for the recreational runner. It may be, for instance, that interval training is associated with race time, but does not help predict time for a longer race on the basis of time for a shorter race, because interval training improves velocity at both distances. Factors associated with race time and race time prediction are related but quite distinct scientific questions. For instance, a user might be asked to enter the time of a recent 10 km race in order to predict the time of a forthcoming marathon. Race time predictors are widely available on the Web, and typically predict time of a future race on the basis of previous race of a different distance. A second important question for long-distance runners concerns race time prediction, critical for pacing during the early stages of a race. Modifiable factors, such as training, may suggest changes that a runner might make to improve race times factors that cannot be modified, such as age or sex, can be used to make fair comparisons between different runners. One key question for such runners concerns factors associated with performance.

Many millions of recreational runners compete in long-distance races each year. Our findings can be used to inform race training and to provide more accurate race time predictions for better pacing. The mean squared error for Riegel was 381 compared to 228 (model based on one prior race) and 208 (model based on two prior races). We built two models to predict marathon time. The commonly used Riegel formula for race time prediction was well-calibrated for races up to a half-marathon, but dramatically underestimated marathon time, giving times at least 10 min too fast for half of runners. Tempo runs were more strongly associated with velocity for shorter distances, while typical weekly training mileage and interval training had similar associations with velocity for all race distances. The difference in velocity between males and females decreased with increasing distance.


Sex, age, BMI and race training were associated with mean race velocity for all race distances.
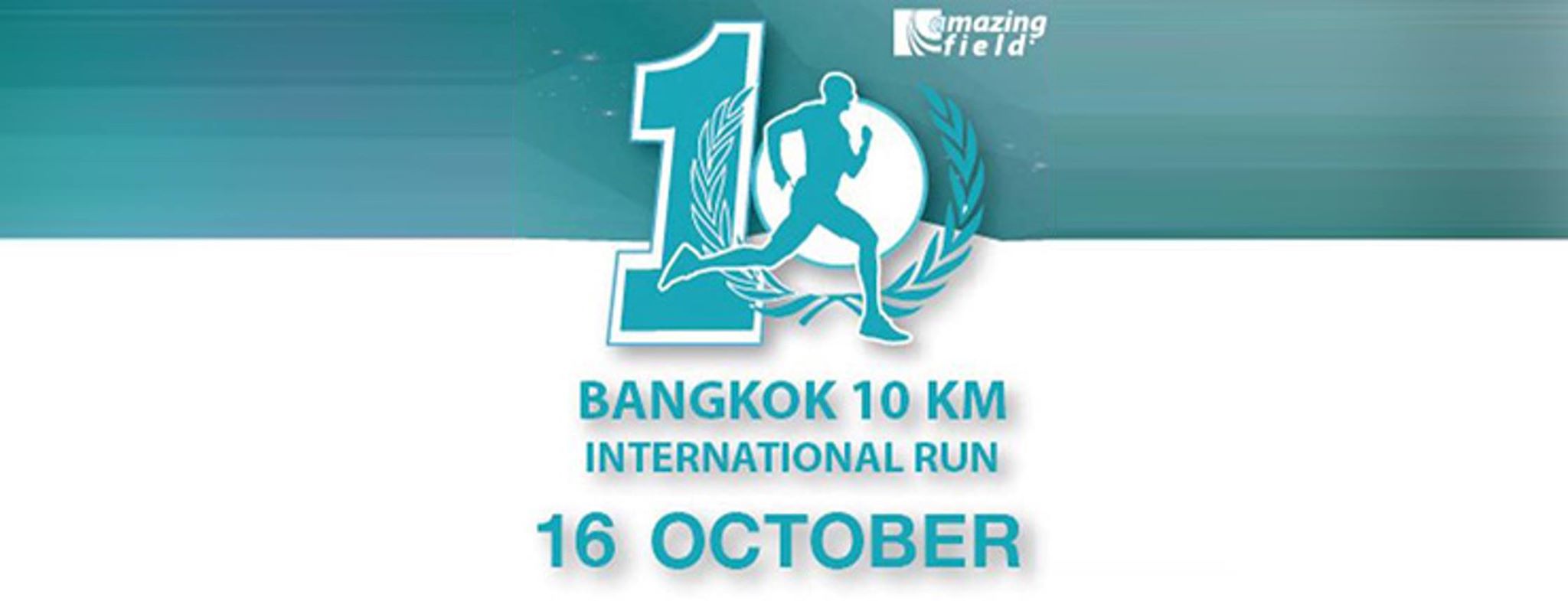
The cohort was split 2:1 into a training set and validation set to create models to predict race time. An Internet survey was used to collect data from recreational endurance runners ( N = 2303). We examined factors associated with race performance and explored methods for race time prediction using information routinely available to a recreational runner. Studies of endurance running have typically involved elite athletes, small sample sizes and measures that require special expertise or equipment.
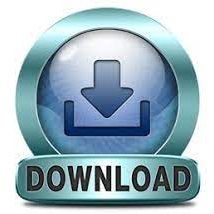